In the second episode in our series on foundation models for science, we discuss Oak Ridge National Laboratory’s work and hear about lessons learned from the recent 1000 Scientists AI Jam, a recent event that brought together researchers from several Department of Energy national laboratories, OpenAI and Anthropic. My guest is Prasanna Balaprakash, ORNL’s director of AI programs. We talk about how foundation models could help with climate forecasts and his team’s 2024 Gordon Bell finalist research and futuristic work that applies principles of swarm intelligence for managing distributed computing resources.
You’ll meet:
- Prasanna Balaprakash has been the director of artificial intelligence programs at Oak Ridge National Laboratory (ORNL) since March 2023. Previously he had worked as a postdoctoral researcher and staff computer scientist at Argonne National Laboratory. He was a 2018 recipient of a Department of Energy Early Career Research Program award.
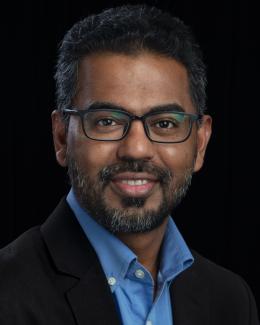
From the episode:
Prasanna mentioned laboratory-directed research & development (LDRD) funding that supports the AI initiatives he discussed. Researchers at ORNL and other D.O.E. national laboratories can apply for these lab-based funds to support cutting-edge projects.
Oak Ridge Leadership Computing Facility houses the Frontier supercomputer, which came online in 2022 and was the first exascale computer on the TOP500 list.
Prasanna described two foundation models that he and his Oak Ridge National Laboratory are working on:
- ORBIT, the Oak Ridge Based Foundation Model for Earth System Predictability is based on data from large-scale climate simulations, such as those from the Department of Energy’s Energy Exascale Earth System model (E3SM). Prasanna mentioned the recent high-resolution modeling work, SCREAM, that won the 2023 ACM Gordon Bell Special Prize for Climate Modeling. The ORBIT team was recognized as a finalist for the 2024 award.
- SWARM: Scientific Workflow Applications on Resilient Metasystem applies ideas from swarm intelligence toward the management of distributed computing resources. It’s funded by the Department of Energy’s Advanced Scientific Computing Research (ASCR, pronounced os-kar) program.
We also discussed the 1000 Scientists AI Jam, held on February 28, 2025, at nine DOE laboratories including ORNL in partnership with OpenAI and Anthropic. One of the tools researchers used during the jam was OpenAI’s Deep Research.
Prasanna mentioned how hardware intersects with new developments in AI and mentioned work on AI accelerators by Groq, Cerebras and SambaNova.
Related episodes:
- Ian Foster: Exploring and Evaluating Foundation Models
- Anil Ananthaswamy: AI’s Nobel Moment
- Paulina Rodriguez: Building Credibility and Authenticity
Featured image (flock of birds) by Christoffer A Rasmussen (Rasmussen29892 at da.wikipedia) – Own work, Public Domain,
Transcript
Sarah Webb 00:00
On Science in Parallel, we’ve launched a series about artificial intelligence and science focusing on foundation models. These tools resemble the large language models that support chat bots like ChatGPT in research, they could help scientists analyze complex data, integrate information from different sources and develop new hypotheses. I’m your host, Sarah Webb, in this episode, we’ll focus on Oak Ridge National Laboratory’s work on foundation models, including examining earth systems and applications of swarm intelligence. We’ll also discuss the recent 1000 Scientist AI Jam at the Department of Energy National Laboratories in partnership with OpenAI and Anthropic.
Prasanna Balaprakash 01:04
I’m Prasanna. Balaprakash. I’m the director of AI programs at Oak Ridge National Lab.
Sarah Webb 01:09
Prasanna, it is great to have you on the podcast.
Prasanna Balaprakash 01:13
Thank you, Sarah, thanks for having me.
Sarah Webb 01:15
So tell me about that job. I mean, that sounds like that could involve a lot of different responsibilities.
Prasanna Balaprakash 01:22
It is really an exciting job. It’s a lab-wide crosscut research initiative focused on developing secure, trustworthy and efficient AI solutions for advancing scientific discovery, modeling complex systems, optimizing complex systems, and to really bring AI to the experimental facilities where we can accelerate the experiments. So that’s sort of into two axises. The one is the application axis that I was talking about. You know, it sort of covers almost the entire DOE spectrum, because Oak Ridge has such a diverse research portfolio, right? So starting from computing, where we have lot of advanced AI scaling methods and optimization methods and so on, so forth, but lot of applications in the environmental science, biology, material science, neutron science and fission and fusion energy and national security.
Speaker 1 02:14
AI is everywhere, but the role of the AI initiative here is identify major challenges, like long term challenges and develop foundational methods that spans these secure, trustworthy and efficient AI solutions and serve the applications that are already there across the DOE and across ORNL. So to that end, it’s what we call lab director research and development. So it’s an investment from the lab looking at various types of these technologies, and say, Okay, we set the direction for this initiative and bring proposals and fund proposals on and really advance various areas of secure, trustworthy and efficient AI solutions.
Sarah Webb 02:57
It sounds like foundation models would be a piece of that. Can you talk a little bit about how that area is emerging in the midst of this work on AI?
Prasanna Balaprakash 03:08
The foundation model is an important area for us. In fact, this is one of the major thrusts within ORNL’s AI initiative. So before talking about foundation models, so what was the sort of business-as-usual? The business-as-usual is we take a data and we develop AI models and data-driven AI machine learning models for that particular data set, or for this particular experiment, or for the particular application. Foundation models are really changing the game. So foundation models take roots and inspiration from how the language models work, right? So typically, in language model setting, the foundation is take a lot of internet data, and all you do is train the model that can predict the next word in a sentence, right? That’s a base, okay, and then you take that model and fine-tune that for chat application. That’s where you know, the OpenAI, ChatGPT and other kinds of chat applications come in. The key advantage here is that you are not training a model specific to one data set. Here, you train this model on very large data, this internet data, and then you can go and fine-tune on various types of applications. And there are a lot of advantages to that.
Prasanna Balaprakash 04:17
So one of the things that we see is the emergent phenomena, which is not in the training data. But when you use the foundation model, that is trained on large amounts of data, you start to see amazing capabilities, problem-solving capabilities coming out of these models. Now, we are trying to do similar things for science applications, right? So we have large amounts of scientific data. In fact, DOE is one of the leading producers of diverse scientific data in the world: simulation data, experimental data, lot of scientific papers. And even within each of these modalities, you have different areas. Our goal here is to begin with, we are focusing on domains, so to speak. Let’s say take computational fluid dynamics. It’s one of the key areas for DOE because many physical phenomena can be modeled as computational fluid dynamics. And the traditional way to do that is you have to do very large-scale simulation. And this has applications in airplane design, wing design, nuclear reactor design, fusion, fission and so on.
Prasanna Balaprakash 05:19
Typically to do this, we need large computing power. But the good thing is, we have large amount of data available within the DOE complex. Now we are taking all this computation, fluid dynamics, data and train a foundation model, right, which can then be taken and fine-tuned for specific applications, right? And that is a big advantage, because in many applications, we don’t have lot of data, right, because simulations are expensive, but if we bring together all these data and train a foundation model and take that model to fine tune on reactor design for fusion and fusion reactors and aerowing design and so on so forth, each application, they don’t have to have lot of data. But given this base model, we can quickly adapt this foundation model on these specific applications. So this is transformative.
Sarah Webb 06:10
What are the challenges in doing this? Because this is coming at the problem from a different perspective.
Prasanna Balaprakash 06:17
Yeah, there are challenges at every stage. That’s why we are doing it.
Sarah Webb 06:21
That’s what makes it fun. I’m sure,
Prasanna Balaprakash 06:22
A lot of fun, but as I said, we have lot of understanding on how to do language model thanks to all the advancements in the industry and the software stack and whatnot. But when it comes to our foundation model for science, we are just getting started. We are making lot of progress across the DOE complex, but how to represent molecules and materials as what we call tokens. in a language model. Tokens in a language model, it’s pretty simple. One word is the basic unit of information, right? So this itself is up for grabs, right when it comes for training foundation models for our science applications that span across multiple modalities, that span across scientific literature, simulation data, experimental data, and so on, so forth. So now we are talking about at least 15-20 different modalities, even for a one particular domain, right? Here we are talking about graphs, language, images, and images in different formats, because different experimental characterization has different types of images. So that itself is very, very complex and very challenging to think through. How can we tokenize them?
Prasanna Balaprakash 06:25
If you take a language as a sequence, it’s a one-dimensional sequence, right? You can preprocess them in different ways, but essentially the underlying structure is one-dimensional sequence. Science problems are very, very high dimensional, right? So you take a multivariate time series. Computational fluid dynamics, it has time space and N number of variables that you need to evolve over time and space. And often they are not uniform, but they are very irregular, and so on so forth. So there are so many different things. Now think about the complexity, right? So one dimensional sequence to the N dimensional sequence where N is very high, right? So how can we develop efficient models to that end? How can we think about model architectures? Because we have to start from somewhere, but essentially, we really have to rethink how to develop large model architecture that can capture all this information in a meaningful way.
Prasanna Balaprakash 08:31
And training means we need large computing resources. And even though I’m sitting 50 feet from the Frontier supercomputer, I cannot just go and train the model whenever I want. We had to get an allocation, like anyone else in the U.S. or the research community, right? So getting access to compute resources where we can train large models for a long time and again, most of the language models are optimized for NVIDIA platform, so GPUs, whereas Frontier is AMD, so we have to really think about how to optimize these models on different platforms. The computational fluid dynamics, the simulations, they have structure. They follow physical processes in nature. It’s not random. So how to ensure that physical consistency outside the training data regime? It doesn’t go and say, have a temperature of million degrees on planet Earth. That’s physically impossible, right, at least on the surface. So we incorporate physics as part of the model architecture and also as part of the training to ensure these kinds of physical consistencies. So that is one part of the trustworthiness.
Prasanna Balaprakash 09:33
And then, even if you have trained all these things, when it comes to science, you can predict something, but then how to ensure that the prediction is trustworthy. So what we do is we incorporate uncertainty quantification techniques into these models to ensure that the model not only give you prediction, but also a bound associated with that prediction so that when the bound is very large, you know that you cannot trust the model prediction. Whereas when the bound is very, very small. The model is sort of confident, right? Doing this on a classical machine learning algorithm and whatnot, it’s relatively easy, but doing this on a foundation model with billions of parameters, it’s much, much harder. Then how can we validate these models? Verify these models? Because we cannot just open up the model and understand what is happening inside. It’s impossible because you are dealing with billions of parameters inside the model. Those are all very open challenges.
Sarah Webb 10:25
It sounds like you’re learning how to build it as you build it.
Prasanna Balaprakash 10:29
Absolutely. You summarized it well, and we are just getting started.
Sarah Webb 10:33
Well, let’s talk about a couple examples. Let’s start with ORBIT, the Oak Ridge-Based Foundation Model for Earth System Predictability. Modeling climate systems is something that DOE has been doing in various ways for a long time. So talk a little bit about what ORBIT does and how that’s different from the traditional way of looking at earth systems.
Prasanna Balaprakash 10:58
The traditional way of doing this is very large-scale simulation, right? The 2023 Gordon Bell for the climate track went to a team who simulated specific aspects of the Earth system on almost the entire Frontier.
Sarah Webb 11:13
That simulation using a version of the Energy Exascale Earth System Model known as E3SM could simulate realistic cloud formations across the globe over 15 months with each day of compute time,
Prasanna Balaprakash 11:29
It takes lot of compute resources to simulate one day of the Earth. You have so many different components. You have so many variables. Now think about this: If we can generate a surrogate model for that simulation and can run the simulation using this foundation model in a matter of minutes on a laptop. So this particular work, what we did is we have large amount of simulation data from different parameterizations, different assumptions and different models. So we took all that data and trained a very large-scale model. So it’s a very high dimensional data as well. So we had to adapt and optimize the model architecture on Frontier. And we trained this model, and very, very fast, we exceeded the exaflop as a sustained throughput in training. This is the first time that foundation model that was developed for an AI system exceeded the exaflop. There are several innovations we had to do that on the data side, on the model, architecture side, optimization side, and so on so forth. And the team was nominated for Gordon Bell finalist in 2024 to recognize those innovations. So we take all the simulation data, train a model, and then take that model and fine-tune that for various types of system predictability tasks, temperature, pressure, how this evolves, and so on, so forth, right? But now the value is, we can do this really, really fast, right? So if you have a set of fine-tuning tasks already, then you can do this sort of forecast within seconds. It doesn’t mean that we don’t need the simulator, E3SM, and other kinds of things are super, super-important because, you know, they produce this data. But then a foundation model that is trained on various types of simulation data can act as a surrogate for the simulation. Now people don’t need to use supercomputers to do those forecasts.
Sarah Webb 13:25
It’s faster, cheaper and more energy efficient than large scale simulations. It also means that individual scientists without access to large supercomputers like Frontier can ask questions and make initial forecasts based on them.
Speaker 1 13:41
But it’s also democratizing, having these types of models, people can take it and fine tune on their own, own data sets, and now you have a very powerful model, even though, if you don’t have lot of data, this model can do reasonably well. And if you have more data, then this model can do really, really well.
Sarah Webb 13:59
Another project Prasanna and his team are working on is called SWARM, which stands for the Scientific Workflow Application for Resilient Metasystems. The work connects back to some of his grad school interests. Then he was thinking about how groups in nature, such as ant colonies or fish schools, make decisions and act collectively, and how those principles could be used in computing.
Speaker 1 14:24
The SWARM project, it’s a DOE ASCR-funded project. It’s very futuristic, and this is one of the things that I have been trying to do for a while, swarm intelligence. I want to bring that more to the high-performance computing, and this project was a fantastic opportunity. Let me give you a quick summary. Swarm intelligence is a subfield of artificial intelligence where you develop and design intelligence systems based on swarm behavior, inspired by nature.
Sarah Webb 14:54
We see this behavior with colonies of insects, flocks of birds and schools of fish. As ants search for food, they leave pheromones, tracer molecules, that allow other members of the colony to follow in their footsteps. Over time, more ants follow the trails with higher levels of the tracers, optimizing the shortest path to a meal. Swarm behavior also shows up in flocks of birds, in schools of fish, as they move together, but avoid colliding into each other. No single ant, bird or fish is directing the group.
Speaker 1 15:28
You have simple rules, simple organisms like doing simple things, but at a macroscopic level, you get something amazing, like fault tolerance and cooperation and various kinds of things, you know, moving away from danger. Fish schools are notorious for that, right? They always tend to be in a big school to confuse the predator, and even if some of them get eaten, the others can escape and so on and so forth. So there’s a lot of this, this resiliency built in this nature, right? But if you go and take look at the systems that we are building, especially high-performance computing systems, take a simple example. Simple example of how to submit the job in a big supercomputer. It’s a centralized scheduler, and if someone wants to run a job in different places, it’s up to the user to go and figure out where to run the job, how to run the job, and so on so forth.
Prasanna Balaprakash 16:17
Now let’s change the paradigm. Instead of a user submitting the job, the resources will talk to each other and automatically figure out where to run the job and how to run the job. And if some of the resources fail, let’s say, you know, we are talking about edge resources, high-performance computing resources and whatnot. If some of them fail, their neighbor can sense it and automatically take over the job and execute right? If some node is failing, the neighboring nodes can sort of look into it and help migrate the job to a different place. So we are really thinking about changing the paradigm, right? So moving from all centralized to what happens if you have completely decentralized system where each node in a big supercomputer is sort of aware of its existence and able to monitor and to execute jobs and in a swarm-intelligent way, right? This will provide a lot of the fault tolerance and so on so forth. Those are things that we don’t need to explicitly program.
Prasanna Balaprakash 17:17
One of the key challenges in swarm-intelligent systems: Each agent is not so powerful. They are relatively less intelligent. It doesn’t have a lot of capabilities. But now, thanks to large language models and reasoning models, think about these swarm intelligent agents that are powered by advanced reasoning models, we are sort of empowering each agent in the swarm intelligence system with this advanced reasoning capability from the large language models, each agent now talks to each other in plain English. I’m doing this. What are you doing? Okay, I have a job like this. Can you take it over? And then he said, Okay, my resource capacity is this much, so I cannot run it now. Okay, you go and talk to someone like so we can enable that sort of communication across the agents and swarm-intelligence-based resilience systems to solve some of the major problems in distributed infrastructure across the DOE complex,
Sarah Webb 18:17
A scale question: At this point, how big is your swarm?
Prasanna Balaprakash 18:21
We are doing a lot of simulations. So right now we are in the design phase, and we are developing consensus algorithms between the agents how to do automated addition making right so without any centralized controller. We are also like studying various types of okay, if this one fails and if you increase the failure rate, what are all the various kinds of behaviors that we see and so on so forth. We cannot go and break a node in the supercomputer. So that’s why we do a lot of simulation studies, benchmarking, developing new algorithms, bringing the reasoning model capabilities into this, into these agents and whatnot. We are also having a test bed to test these things so where we can sort of emulate the failures.
Prasanna Balaprakash 19:03
So right now we are doing this at a small scale, like less than 100 agents, but our goal is really looking 30 years from now, how do you look like right? So really distributed capabilities, experimental facilities, sensors, drones and so on, so forth. Now think about each one of them is sort of have this reasoning capability, and they sort of collaborate and cooperate and send messages to each other. Oh, you know, I’m collecting data here, where to send. Oh, don’t send it to me, because I’m already occupied with something but send it to some other place. So we are really envisioning a very futuristic distributed infrastructure. And scaling question is important, right? How we can scale important, right? How we can scale this? Not to 1000, not to 10,000, but hundreds of thousands of agents, because I think that will really push the state of the art and opens up a lot of interesting questions.
Sarah Webb 19:53
Obviously, private industry is doing a lot of work in this space. What do you see as industry’s role versus the national labs’ role. How might they work together?
Prasanna Balaprakash 20:02
This is an exciting area, especially the collaboration between industry and national labs along with academia. There are various ways that national labs and industry can work together. In fact, we are already doing it. So if you look at what industry is doing, to a large extent, they are developing language models and improving the capabilities of the reasoning models. They’re primarily focused on business use cases, areas where you can make money next year or next six months, and in fact, these models are often trained on benchmarks to show their capabilities and whatnot, right? But here is the thing, so what we are doing is taking that language model and incorporating that in the scientific discovery workflow. And that is something that they didn’t think about until we showed Hey, these are the ways that we are using the language model to advance scientific discovery. That was like, fascinating for them.
Prasanna Balaprakash 20:54
They want to know where the models are good, where the models are not good, right? So these models are not 100% foolproof, and the science community can provide tougher and tougher benchmarks. And this will help the industry to solve harder problems, which will eventually help them to do better in various types of tasks. I was talking about the physical consistency, right, which is such an important thing for us, because we have a lot of domain knowledge and lot of data from scientific simulations. We are not competing with the industry, but we are finding ways to bring what they are doing to our and looking at, let’s say, the graph models for material discovery, ORBIT-type models for earth system predictability. So these are different modalities. These are not language modalities. We are leveraging our industry advancements and bringing that to the scientific discovery workflow and developing other types of foundation models which industry is not looking at, but we see all these things coming together for accelerating scientific discovery.
Sarah Webb 21:50
So I want to talk to you more about this 1000 Scientists AI Jam session that recently happened across the DOE labs. I believe it was February 28.
Prasanna Balaprakash 22:00
Correct.
Sarah Webb 22:01
First of all, can you set the scene at Oak Ridge for me? How many people there were involved? This wasn’t just in your backyard. It was across the lab system.
Sarah Webb 22:10
Although we called it the 1000 Scientists Jam, and at the end of the day, we had 1400 scientists coming together across nine national labs in a single day to explore what the frontier AI models from industry, especially OpenAI and Anthropic. They were the partners in the jam, and they provided access to the models. And 1400 scientists came together, and they brought their science problem and see how the frontier models can help them to solve those problems. So that’s an exciting and a very interesting partnership model. We have been partnering with hardware vendors, for example, AMD and Intel, NVIDIA for building machines. So that was one of our major partnership especially on the computing side. But this is the first time we said, Okay, this is a this is a very good model for for collaboration between industry and and national labs. And as I said, national labs, we have simulation, we have experimental facilities, and we build other types of foundation models, right? How can we bring the reasoning model capabilities into scientific workflows and combine science foundation models with reasoning model, language model. So there are a lot of interesting things that we can do together and OpenAI was excited. People here at Oak Ridge and across the nine national labs, we had a very, very productive day exploring the capabilities of these models.
Sarah Webb 23:34
How many people at Oak Ridge were involved?
Prasanna Balaprakash 23:36
Eighty.
Sarah Webb 23:38
Can you give any examples of something that either was interesting or surprising in terms of the types of problems that you were thinking about?
Prasanna Balaprakash 23:47
So the group of people who worked on nuclear reactor design, so they were starting with, okay, we want to design these type of reactors. What are the design sizes need to do? And OpenAI provided the bleeding edge model without any rate limitations. So basically, we can ask as many as questions we want. So starting from this high-level question, okay, I want to design a reactor with this type of efficiency and with this kind of output. How can I design this? So it started with these assumptions and provided ideas how you can do like this with this sort of material for the reactor. It was a pretty interesting exercise of how one can design new materials for reactors. So that’s an interesting one.
Prasanna Balaprakash 24:28
We also had someone who was designing materials for a battery. So we also had access to what we call Deep Research, you probably might have heard, from OpenAI. Deep Research is a capability within this model that can go glean around the internet and come up with all sort of information and summarize them and can provide you information. What is the state of the art? What are the open questions? What are the things that you need to look at, and whatnot? So it takes 20 minutes, but that’s how people started. In the morning, we asked them go and do a Deep Research on the problem that you want to do. In 10, 20 minutes, it came up with a 20-25, page summary of basically what the state of the art is. It’s not 100% correct, but you get a lot of information in such a short time.
Prasanna Balaprakash 25:11
So for the battery materials, again, is like, we’re exploring various things. And this person came out and said, like, hey, the kind of work that I did in one afternoon, it will take three months for me to do this. That’s the other facet of it. Like, how can we do a lot of this work that includes the Deep Research, code generation, running that code on the computer, getting the results and putting it back? So there’s a lot of modalities that went into this model. So that’s another big takeaway, right? So how we can reduce the time needed to do various facets of research? So that’s another thing. And we also explored how to address I/O problems in big super computers. What are the ways depending on the workloads and whatnot? So what are the ways that we can do this? So these are some of the examples.
Prasanna Balaprakash 25:57
There are other things related to material science, improving the security of the electric grid, or what are the ways that we can do that? And different aspects of biological research, like how to analyze the plant phenotype data, extract features. So they came up with the dataset; they used the model to write code and analyze the data and give this analysis results back, and generated hypothesis what this means. So a lot of interesting things came out. And also there are people who came out with: The physical consistency is totally off. This is not the right result. The model is not good in these deep technical areas and so on. So it was a very good learning experience for all of us.
Sarah Webb 26:34
Is there anything else that you think is important to mention about those caveats? And you were talking about security, reliability.
Prasanna Balaprakash 26:39
We talked about reasoning. In many cases, it’s not just predictive accuracy, right? So often people ask why, answering the why question rather than, okay, I give this structure, this is a prediction, right? So why? That is science, and we see the traces of it with respect to language model on generic tasks. But really, we have to make advancements in that space. So given the data and we can explain why this physical process, what is the underlying equations that determine this physical process? We often have sort of a first order approximation of the physical process. But what are the second, third, fourth, fifth order? Or is there another set of equations that can explain this in a better way right now. You know you have a machine that can keep on trying different possibilities, 24/7 and think about scaling that. So those are things like what we call causal reasoning. Again, I want to stress the uncertainty quantification aspect, validation and verification aspect and efficient AI, right? So we are looking into how to make these trainings much more efficient, specifically. So yes, we do on large supercomputers, but also there is new emerging computing paradigm like accelerators such as SambaNova, Cerebras and Groq and others.
Prasanna Balaprakash 27:50
So they’re primarily looking at language models, but there’s a lot of potential to also run for science foundation models and so on so forth, right? So those are some of the areas that we had to figure out new computing paradigm, an efficient computing paradigm. And it’s not that one solution will fit all, but it’s going to be heterogeneous. So what are, what are the right set of hardware for right set of models? And there are a lot of fundamental applied math and computer science questions that still need to be answered, deep understanding of these large models. How can we humans understand these models? It’s an open question. How can we ensure that the model understands the physics, as opposed to purely data-driven? So that is a grand challenge. That’s a very hard thing, but we have to make progress on it.
Prasanna Balaprakash 28:39
What are the math challenges that we have and how can we build powerful models with small amount of data? Even in the language models, if you carefully select the training data, you can fine-tune the model for reasoning with 1000 examples. You don’t need millions and millions of examples. So those are some early results that gives us confidence that we can do this better. And so these are applied math challenges, computer science challenges, right? So how to do codesign, how to do efficient algorithms for different hardware, and bring together the traditional computer science that we have in DOE, the computational science, and mix that with the AI. What are the foundational challenges, fundamental challenges? So those are things that we need to address especially when it comes to computational science, right? So there are many fundamental applied math and computer science challenges that we need to overcome, and those are sort of long term. So we need to keep on investing on these foundational aspects of building AI systems.
Sarah Webb 29:38
So in other words, we’re far from done.
Prasanna Balaprakash 29:40
We are so far from done, but progress is amazing. That’s the important thing to notice, especially in the language modeling side. But we need to translate that to the scientific foundation models. That needs really a large, coordinated effort across the DOE complex, bringing industry and academia together. So there are a lot of important science questions that we can answer by working together with industry and academia.
Sarah Webb 30:04
Prasanna, thank you so much. It’s been such a pleasure.
Prasanna Balaprakash 30:09
Thank you, Sarah, and it was a great conversation.
Sarah Webb 30:12
To learn more about Prasanna Balaprakash, the ORBIT and SWARM projects, and the 1000 Scientists. AI Jam, check out our show notes at scienceinparallel.org.
Science in Parallel is produced by the Krell Institute and is a media project of the Department of Energy Computational Science Graduate Fellowship program. Any opinions expressed are those of the speaker and not those of their employers, the Krell Institute or the U.S. Department of Energy. Our music is by Steve O’Reilly. This episode was written, produced and edited by me, Sarah Webb.
Transcript prepared using otter.ai with some copyediting.